Package: iDINGO 1.0.4
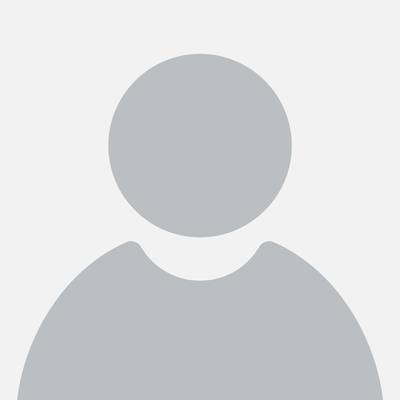
Caleb A. Class
iDINGO: Integrative Differential Network Analysis in Genomics
Fits covariate dependent partial correlation matrices for integrative models to identify differential networks between two groups. The methods are described in Class et. al., (2018) <doi:10.1093/bioinformatics/btx750> and Ha et. al., (2015) <doi:10.1093/bioinformatics/btv406>.
Authors:
iDINGO_1.0.4.tar.gz
iDINGO_1.0.4.tar.gz(r-4.5-noble)iDINGO_1.0.4.tar.gz(r-4.4-noble)
iDINGO_1.0.4.tgz(r-4.4-emscripten)iDINGO_1.0.4.tgz(r-4.3-emscripten)
iDINGO.pdf |iDINGO.html✨
iDINGO/json (API)
NEWS
# Install 'iDINGO' in R: |
install.packages('iDINGO', repos = c('https://cran.r-universe.dev', 'https://cloud.r-project.org')) |
This package does not link to any Github/Gitlab/R-forge repository. No issue tracker or development information is available.
Last updated 5 years agofrom:f080579709. Checks:2 OK. Indexed: yes.
Target | Result | Latest binary |
---|---|---|
Doc / Vignettes | OK | Feb 11 2025 |
R-4.5-linux | OK | Feb 11 2025 |
Exports:dingoextendedBICGreg.emidingoplotNetworkscaledMatscoring.bootscoring.boot.parallelSigmaxsingle.boottrans.Fisher
Dependencies:base64encbslibcachemclicolorspacecpp11digestevaluatefarverfastmapfontawesomefsGGMridgeglassogluehighrhtmltoolshtmlwidgetsigraphjquerylibjsonliteknitrlabelinglatticelifecyclemagrittrMASSMatrixmemoisemimemunsellmvtnormpkgconfigR6rappdirsRColorBrewerrlangrmarkdownsassscalestinytexvctrsviridisLitevisNetworkxfunyaml
Readme and manuals
Help Manual
Help page | Topics |
---|---|
iDINGO: Integrative Differential Network Analysis in Genomics | iDINGO-package iDINGO |
Modified TCGA Breast Cancer data | brca |
Fit DINGO model | dingo |
Extended bayesian information criteria for gaussian graphical models | extendedBIC |
Modified TCGA Glioblastoma data | gbm |
Fitting precision regression models | Greg.em |
Fit iDINGO model | idingo |
Plot differential network | plotNetwork |
scale a square matrix | scaledMat |
Calculating differential score | scoring.boot |
Calculating differential score with parallel bootstrap scoring | scoring.boot.parallel |
group specific covariance matrices | Sigmax |
Calculating differential score for a single bootstrap | single.boot |
Fisher's Z-transformation | trans.Fisher |