Package: apc 2.0.0
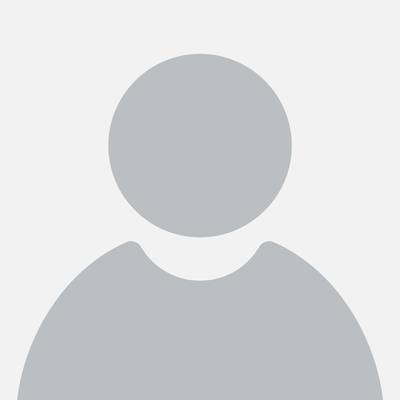
Bent Nielsen
apc: Age-Period-Cohort Analysis
Functions for age-period-cohort analysis. Aggregate data can be organised in matrices indexed by age-cohort, age-period or cohort-period. The data can include dose and response or just doses. The statistical model is a generalized linear model (GLM) allowing for 3,2,1 or 0 of the age-period-cohort factors. Individual-level data should have a row for each individual and columns for each of age, period, and cohort. The statistical model for repeated cross-section is a generalized linear model. The statistical model for panel data is ordinary least squares. The canonical parametrisation of Kuang, Nielsen and Nielsen (2008) <doi:10.1093/biomet/asn026> is used. Thus, the analysis does not rely on ad hoc identification.
Authors:
apc_2.0.0.tar.gz
apc_2.0.0.tar.gz(r-4.5-noble)apc_2.0.0.tar.gz(r-4.4-noble)
apc_2.0.0.tgz(r-4.4-emscripten)apc_2.0.0.tgz(r-4.3-emscripten)
apc.pdf |apc.html✨
apc/json (API)
NEWS
# Install 'apc' in R: |
install.packages('apc', repos = c('https://cran.r-universe.dev', 'https://cloud.r-project.org')) |
This package does not link to any Github/Gitlab/R-forge repository. No issue tracker or development information is available.
Last updated 4 years agofrom:94a277f1b5. Checks:OK: 1 NOTE: 1. Indexed: yes.
Target | Result | Date |
---|---|---|
Doc / Vignettes | OK | Aug 23 2024 |
R-4.5-linux | NOTE | Aug 23 2024 |
Exports:apc.data.listapc.data.list.subsetapc.data.sumsapc.fit.modelapc.fit.tableapc.forecast.acapc.forecast.apapc.forecast.apcapc.get.designapc.get.design.collinearapc.get.indexapc.identifyapc.indiv.compare.directapc.indiv.design.collinearapc.indiv.design.modelapc.indiv.est.modelapc.indiv.estimate.TSapc.indiv.fit.modelapc.indiv.logit.TSapc.indiv.LRtableapc.indiv.LRtable.TSapc.indiv.LRtest.fullapcapc.indiv.LRtest.TSapc.indiv.model.tableapc.indiv.waldtableapc.indiv.waldtable.TSapc.indiv.waldtest.fullapcapc.indiv.waldtest.TSapc.internal.function.date.2.characterapc.plot.data.allapc.plot.data.levelapc.plot.data.sparsityapc.plot.data.sumsapc.plot.data.withinapc.plot.data.within.all.sixapc.plot.fitapc.plot.fit.allapc.plot.fit.fitted.valuesapc.plot.fit.linear.predictorsapc.plot.fit.ptapc.plot.fit.residualsapc.polygondata.aidsdata.asbestosdata.asbestos.2013data.asbestos.2013.mendata.asbestos.2013.womendata.Belgian.lung.cancerdata.Italian.bladder.cancerdata.Japanese.breast.cancerdata.loss.BZdata.loss.TAdata.loss.VNJdata.loss.XLdata.RH.mortality.dkdata.RH.mortality.nodata.US.prostate.canceris.trianglenew.apc.identifynew.apc.plot.fittriangle.cumulativetriangle.incrementalvector.2.triangle
Dependencies:abindactuarAERbackportsbdsmatrixbiglmbootbroomcarcarDataChainLadderclicodacollapsecolorspacecowplotcplmcpp11DBIDerivdigestdoBydplyrexpintfansifarverFormulagenericsggplot2gluegtableISLRisobandlabelinglatticelifecyclelme4lmtestmagrittrMASSMatrixMatrixModelsmaxLikmgcvmicrobenchmarkminqamiscToolsmitoolsmodelrmunsellnlmenloptrnnetnumDerivpbkrtestpillarpkgconfigplmplyrpurrrquantregR6rbibutilsRColorBrewerRcppRcppArmadilloRcppEigenRdpackreshapereshape2rlangsandwichscalesSparseMstatmodstringistringrsurveysurvivalsystemfittibbletidyrtidyselecttweedieutf8vctrsviridisLitewithrzoo
Generating new models from design matrix function
Rendered fromNewDesign.Rnw
usingutils::Sweave
on Aug 23 2024.Last update: 2020-10-01
Started: 2015-04-12
Identification: illustrate and check identification used in plot fit function
Rendered fromIdentification.Rnw
usingutils::Sweave
on Aug 23 2024.Last update: 2020-10-01
Started: 2015-04-12
Illustrate and check identification used in plot fit function
Rendered fromReproducingMMNN2015.Rnw
usingutils::Sweave
on Aug 23 2024.Last update: 2020-10-01
Started: 2020-10-01
Introduction: analysis of aggregate data
Rendered fromIntroductionAggregateData.rnw
usingutils::Sweave
on Aug 23 2024.Last update: 2020-10-01
Started: 2020-10-01
Introduction: analysis of individual data
Rendered fromIntroductionIndividualData.rnw
usingutils::Sweave
on Aug 23 2024.Last update: 2020-10-01
Started: 2020-10-01
Introduction: analysis of individual data: further examples
Rendered fromIntroductionIndividualDataFurtherExamples.rnw
usingutils::Sweave
on Aug 23 2024.Last update: 2020-10-01
Started: 2020-10-01
Reproducing HN2016
Rendered fromReproducingHN2016.Rnw
usingutils::Sweave
on Aug 23 2024.Last update: 2020-10-01
Started: 2020-10-01
Reproducing KN2020
Rendered fromReproducingKN2020.Rnw
usingutils::Sweave
on Aug 23 2024.Last update: 2020-10-01
Started: 2020-10-01
Reproducing MMNN2016
Rendered fromReproducingMMNN2016.Rnw
usingutils::Sweave
on Aug 23 2024.Last update: 2020-10-01
Started: 2020-10-01