Package: FlexVarJM 0.1.0
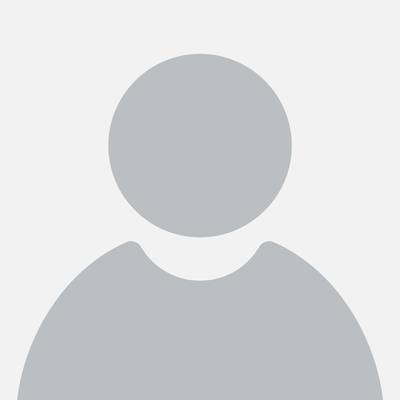
Léonie Courcoul
FlexVarJM: Estimate Joint Models with Subject-Specific Variance
Estimation of mixed models including a subject-specific variance which can be time and covariate dependent. In the joint model framework, the package handles left truncation and allows a flexible dependence structure between the competing events and the longitudinal marker. The estimation is performed under the frequentist framework, using the Marquardt-Levenberg algorithm. (Courcoul, Tzourio, Woodward, Barbieri, Jacqmin-Gadda (2023) <arxiv:2306.16785>).
Authors:
FlexVarJM_0.1.0.tar.gz
FlexVarJM_0.1.0.tar.gz(r-4.5-noble)FlexVarJM_0.1.0.tar.gz(r-4.4-noble)
FlexVarJM_0.1.0.tgz(r-4.4-emscripten)FlexVarJM_0.1.0.tgz(r-4.3-emscripten)
FlexVarJM.pdf |FlexVarJM.html✨
FlexVarJM/json (API)
NEWS
# Install 'FlexVarJM' in R: |
install.packages('FlexVarJM', repos = 'https://cloud.r-project.org') |
Bug tracker:https://github.com/leoniecourcoul/flexvarjm/issues
- Data_toy - Data_toy
Last updated 1 years agofrom:ae1340b859. Checks:3 OK. Indexed: no.
Target | Result | Latest binary |
---|---|---|
Doc / Vignettes | OK | Mar 15 2025 |
R-4.5-linux-x86_64 | OK | Mar 15 2025 |
R-4.4-linux-x86_64 | OK | Mar 15 2025 |
Exports:goodness_of_fitlsjmlsmmpredyn
Dependencies:abindbackportsbootbroomcarcarDataclicodetoolscolorspacecommonmarkcorrplotcowplotcpp11curldata.tableDerivdoBydoParalleldplyrevaluateexactRankTestsfansifarverforeachFormulagenericsggplot2ggpubrggrepelggsciggsignifggtextgluegridExtragridtextgtablehighrisobanditeratorsjpegkm.ciKMsurvknitrlabelinglatticelcmmlifecyclelme4magrittrmarkdownmarqLevAlgMASSMatrixMatrixModelsmaxstatmgcvmicrobenchmarkminqamodelrmunsellmvtnormnlmenloptrnnetnumDerivpbkrtestpillarpkgconfigpngpolynompurrrquantregR6randtoolboxrbibutilsRColorBrewerRcppRcppArmadilloRcppEigenRdpackreformulasrlangrngWELLrstatixscalesspacefillrSparseMstringistringrsurvivalsurvminersurvMisctibbletidyrtidyselectutf8vctrsviridisLitewithrxfunxml2xtableyamlzoo
Citation
To cite package ‘FlexVarJM’ in publications use:
Courcoul L, Barbieri A, Jacqmin-Gadda H (2023). FlexVarJM: Estimate Joint Models with Subject-Specific Variance. R package version 0.1.0, https://CRAN.R-project.org/package=FlexVarJM.
Corresponding BibTeX entry:
@Manual{, title = {FlexVarJM: Estimate Joint Models with Subject-Specific Variance}, author = {Léonie Courcoul and Antoine Barbieri and Hélène Jacqmin-Gadda}, year = {2023}, note = {R package version 0.1.0}, url = {https://CRAN.R-project.org/package=FlexVarJM}, }
Readme and manuals
FlexVarJM
The goal of FlexVarJM is to estimate joint model with subject-specific time-dependent variability.
The global function is ‘lsjm’. It handles to estimate joint model with a marker which has a subject-specific time-dependent variability and competing events with the possibility to take into account the left truncation. For more information you can read the corresponding article : https://arxiv.org/abs/2306.16785
Installation
You can install the development version of FlexVarJM from GitHub with:
# install.packages("devtools")
devtools::install_github("LeonieCourcoul/FlexVarJM")
Example
Estimation
This is an example in a simulated dataset where is a binary variable.
$$y_i(t_{ij}) = \color{blue}\tilde{y}i(t{ij}) \color{black} + \epsilon_{ij} = \beta_0 + b_{0i} + (\beta_1 + b_{1i})t_{ij} + \beta_2 * binary_i + \epsilon_{ij} $$
For the first risk, k = 1, we estimate the following risk function :
$$ \lambda_{i1}(t) = \lambda_{01}(t)\exp(\gamma_{11}*binary_i + \color{blue}\alpha_{11}\tilde{y}i(t) + \color{red}\alpha{\sigma 1} \sigma_i(t) \color{black}) $$ And for the second risk, k = 2 : $$ \lambda_{i2}(t) = \lambda_{02}(t)\exp(\color{blue}\alpha_{21}\tilde{y_i}(t) + \color{blue}\alpha_{22}\tilde{y}'i(t) + \color{red}\alpha{\sigma 2} \sigma_i(t) \color{black}) $$
where :
-
$\epsilon_{i}(t_{ij}) \sim \mathcal{N}(0, \color{red}\sigma_i^2\color{black})$ with $\color{red}\log(\sigma_i(t_{ij})) = \mu_0 + \tau_{0i} + (\mu_1 + \tau_{1i})\times t_{ij} + \mu_2 * binary_i$
-
with $b_i=\left(b_{0i},b_{1i}\right)^{\top}$ and $\tau_i=\left(\tau_{0i},\tau_{1i}\right)^{\top}$ assuming that the two sets of random effects $b_i$ and $\tau_i$ are not independent: $$(b_i, \tau_i)^\top \sim N(0, \Sigma)$$
-
$\lambda_{0k}(t) = \kappa_k^2 t^{\kappa_k^2-1}e^{\zeta_{0k}}$ : Weibull function
-
$\tilde{y}'_i(t)$ is the current slope of the marker $y$
example <- lsjm(formFixed = y~visit+binary,
formRandom = ~ visit,
formGroup = ~ID,
formSurv = Surv(time, event ==1 ) ~ binary,
timeVar = "visit",
data.long = Data_toy,
variability_hetero = TRUE,
formFixedVar =~visit+binary,
formRandomVar =~visit,
correlated_re = TRUE,
sharedtype = c("current value", "variability"),
hazard_baseline = "Weibull",
competing_risk = TRUE,
formSurv_CR = Surv(time, event ==2 ) ~ 1,
hazard_baseline_CR = "Weibull",
sharedtype_CR = c("slope", "variability"),
formSlopeFixed =~1,
formSlopeRandom = ~1,
indices_beta_slope = c(2),
S1 = 500,
S2 = 1000,
nproc = 5,
Comp.Rcpp = TRUE
)
summary(example)
You can access to the table of estimations and standard deviation with :
example$table.res
The computing time is given by :
example$time.compute
The output of the marqLevAlg algorithm is in :
example$result
Finally, some elements of control are in :
example$control
Goodness-of-fit
You can check the goodness-of-fit of the longitudinal submodel and of the survival submodel by computing the predicted random effects :
goodness <- goodness_of_fit(example, graph = T)
Predictions
You can compute the probability for (new) individual(s) to have event 1 or 2 between time s and time s+t years given that he did not experience any event before time s, its trajectory of marker until time s ans the set of estimated parameters. To have a ‘IC%’ confidence interval, the predictions are computed ‘nb.draws’ time and the percentiles of the predictions are computed. For example, for individuals 1 and 3 to experiment the event 1 at time 1.5, 2, and 3, given their measurements until time 1 :
newdata <- Data_toy[which(Data_toy$ID %in% c(1,3)),]
predyn(newdata,example,1, c(1.5,2,3), event = 1, IC = 95, nb.draws = 500, graph = TRUE)
Help Manual
Help page | Topics |
---|---|
Data_toy | Data_toy |
Initialisation of Survival Data at Gauss Kronrod time points | data.GaussKronrod |
Initialisation of Survival Data at Gauss Kronrod time points 2 | data.GaussKronrod2 |
Management of longitudinal data | data.manag.long |
Management of survival data | data.manag.surv |
Management of data for longitudinal submodel | data.time |
Gauss-Kronrod nodes and weights | gaussKronrod |
Predictions for the goodness of fit, of the random effects, the current value for each individuals and the cumulative hazard function for both events | goodness_of_fit |
Initialisation of Longitudinal Submodel | initial.long |
Log-likelihood computation | log_llh |
Log-likelihood computation in RCPP | log_llh_rcpp |
lsjm : Estimation of joint model for longitudinal data with a subject-specific time-dependent variability and time-to-event data. | lsjm |
lsmm : Estimation of location scale mixed model | lsmm |
Predictions computation | pred_s.t.bootstrap.tps |
Predictions computation | pred_s.t.ponctuel.tps |
Dynamic prediction for new individuals | predyn |