Package: stpm 1.7.12
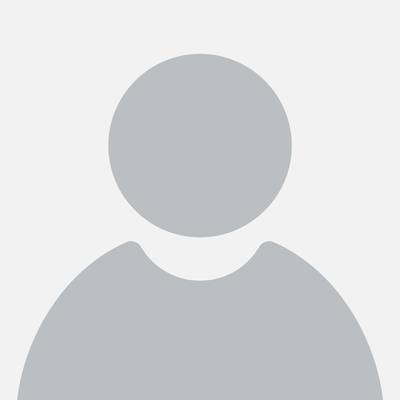
Ilya Y. Zhbannikov
stpm: Stochastic Process Model for Analysis of Longitudinal and Time-to-Event Outcomes
Utilities to estimate parameters of the models with survival functions induced by stochastic covariates. Miscellaneous functions for data preparation and simulation are also provided. For more information, see: (i)"Stochastic model for analysis of longitudinal data on aging and mortality" by Yashin A. et al. (2007), Mathematical Biosciences, 208(2), 538-551, <doi:10.1016/j.mbs.2006.11.006>; (ii) "Health decline, aging and mortality: how are they related?" by Yashin A. et al. (2007), Biogerontology 8(3), 291(302), <doi:10.1007/s10522-006-9073-3>.
Authors:
stpm_1.7.12.tar.gz
stpm_1.7.12.tar.gz(r-4.5-noble)stpm_1.7.12.tar.gz(r-4.4-noble)
stpm_1.7.12.tgz(r-4.4-emscripten)stpm_1.7.12.tgz(r-4.3-emscripten)
stpm.pdf |stpm.html✨
stpm/json (API)
NEWS
# Install 'stpm' in R: |
install.packages('stpm', repos = c('https://cran.r-universe.dev', 'https://cloud.r-project.org')) |
- ex_data - This is the longitudinal genetic dataset.
This package does not link to any Github/Gitlab/R-forge repository. No issue tracker or development information is available.
Last updated 2 years agofrom:66e4b79dc3. Checks:OK: 2. Indexed: yes.
Target | Result | Date |
---|---|---|
Doc / Vignettes | OK | Sep 11 2024 |
R-4.5-linux-x86_64 | OK | Sep 11 2024 |
Exports:prepare_datasim_pobssimdata_contsimdata_discrsimdata_gamma_frailtysimdata_time_depspmspm_con_1dspm_con_1d_gspm_cont_linspm_cont_quad_linspm_continuousspm_discretespm_pobsspm_projectionspm_time_depspm.impute
Dependencies:latticeMASSMatrixnloptrRcppRcppArmadillosas7bdatsurvival