Package: rqPen 4.1.3
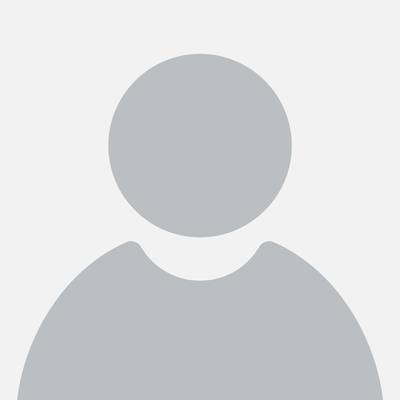
Ben Sherwood
rqPen: Penalized Quantile Regression
Performs penalized quantile regression with LASSO, elastic net, SCAD and MCP penalty functions including group penalties. In addition, offers a group penalty that provides consistent variable selection across quantiles. Provides a function that automatically generates lambdas and evaluates different models with cross validation or BIC, including a large p version of BIC. Below URL provides a link to a work in progress vignette.
Authors:
rqPen_4.1.3.tar.gz
rqPen_4.1.3.tar.gz(r-4.5-noble)rqPen_4.1.3.tar.gz(r-4.4-noble)
rqPen_4.1.3.tgz(r-4.4-emscripten)rqPen_4.1.3.tgz(r-4.3-emscripten)
rqPen.pdf |rqPen.html✨
rqPen/json (API)
NEWS
# Install 'rqPen' in R: |
install.packages('rqPen', repos = 'https://cloud.r-project.org') |
Bug tracker:https://github.com/bssherwood/rqpen/issues
Last updated 2 months agofrom:1e809b9e07. Checks:3 OK. Indexed: no.
Target | Result | Latest binary |
---|---|---|
Doc / Vignettes | OK | Mar 15 2025 |
R-4.5-linux-x86_64 | OK | Mar 15 2025 |
R-4.4-linux-x86_64 | OK | Mar 15 2025 |
Exports:bytau.plotqic.selectrq.gq.penrq.gq.pen.cvrq.group.penrq.group.pen.cvrq.penrq.pen.cv
Dependencies:clidata.tablegluehqreghrqglaslatticelifecycleMASSMatrixMatrixModelsplyrquantregrbibutilsRcppRcppArmadilloRdpackrlangSparseMsurvival
Citation
To cite package ‘rqPen’ in publications use:
Sherwood B, Li S, Maidman A (2025). rqPen: Penalized Quantile Regression. R package version 4.1.3, https://CRAN.R-project.org/package=rqPen.
Corresponding BibTeX entry:
@Manual{, title = {rqPen: Penalized Quantile Regression}, author = {Ben Sherwood and Shaobo Li and Adam Maidman}, year = {2025}, note = {R package version 4.1.3}, url = {https://CRAN.R-project.org/package=rqPen}, }
Readme and manuals
rqPen: Penalized quantile regression
Overview
This R package provides tools for estimating a quantile regression model with a penalized objective function. Implements a variety of penalties, including group penalties.
Installation
For most up to date versions use the following code. However, be warned the github package is often in a state of testing and debugging.
devtools::install_github("bssherwood/rqpen")
The following code will install the more stable CRAN version.
install.packages("rqPen")
Example
library(rqPen)
n<- 200
p<- 30
x0<- matrix(rnorm(n*p),n,p)
x<- cbind(x0, x0^2, x0^3)[,order(rep(1:p,3))]
y<- -2+x[,1]+0.5*x[,2]-x[,3]-0.5*x[,7]+x[,8]-0.2*x[,9]+rt(n,2)
group<- rep(1:p, each=3)
# lasso estimation
# one tau
fit1 <- rq.pen(x,y)
# several values of tau
fit2 <- rq.pen(x,y,tau=c(.2,.5,.8))
# Group SCAD estimation
fit3 <- rq.group.pen(x,y,groups=group,penalty="gSCAD")
# cross validation
cv1 <- rq.pen.cv(x,y)
plot(cv1)
cv2 <- rq.pen.cv(x,y,tau=c(.2,.5,.8))
plot(cv2)
cv3 <- rq.group.pen(x,y,groups=group,penalty="gSCAD")
plot(cv3)
# BIC selection of tuning parameters
qs1 <- qic.select(fit1)
qs2 <- qic.select(fit2)
qs3 <- qic.select(fit3)
See, https://github.com/bssherwood/rqpen/blob/master/ignore/rqPenArticle.pdf, for a vignette. The Huber approach for rq.pen relies on the R package hqreg and work presented in "Semismooth Newton Coordinate Descent Algorithm for Elastic-Net Penalized Huber Loss Regression and Quantile Regression". The Huber approach in rq.group.pen relies on R package hrqglas and work presented in An Efficient Approach to Feature Selection and Estimation for Quantile Regression with Grouped Variables
References
Sherwood, B. and Li, S. (2022) An Efficient Approach to Feature Selection and Estimation for Quantile Regression with Grouped Variables, Statistics and computing, 75.
Yi, C. and Huang, J. (2015) Semismooth Newton Coordinate Descent Algorithm for Elastic-Net Penalized Huber Loss Regression and Quantile Regression, Journal of Computational and Graphical Statistics, 26:3, 547-557.