Package: reportRmd 0.1.1
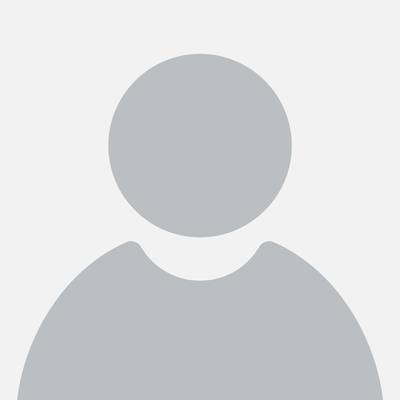
Lisa Avery
reportRmd: Tidy Presentation of Clinical Reporting
Streamlined statistical reporting in 'Rmarkdown' environments. Facilitates the automated reporting of descriptive statistics, multiple univariate models, multivariable models and tables combining these outputs. Plotting functions include customisable survival curves, forest plots from logistic and ordinal regression and bivariate comparison plots.
Authors:
reportRmd_0.1.1.tar.gz
reportRmd_0.1.1.tar.gz(r-4.5-noble)reportRmd_0.1.1.tar.gz(r-4.4-noble)
reportRmd_0.1.1.tgz(r-4.4-emscripten)reportRmd_0.1.1.tgz(r-4.3-emscripten)
reportRmd.pdf |reportRmd.html✨
reportRmd/json (API)
NEWS
# Install 'reportRmd' in R: |
install.packages('reportRmd', repos = c('https://cran.r-universe.dev', 'https://cloud.r-project.org')) |
- ctDNA - Tumour size change over time Longitudinal changes in tumour size since baseline for patients by changes in ctDNA status (clearance, decrease or increase) since baseline.
- pembrolizumab - Survival data Survival status and ctDNA levels for patients receiving pembrolizumab
- uvmodels - Aligning models and parameters
This package does not link to any Github/Gitlab/R-forge repository. No issue tracker or development information is available.
Last updated 2 months agofrom:74456d0333. Checks:3 OK. Indexed: yes.
Target | Result | Latest binary |
---|---|---|
Doc / Vignettes | OK | Mar 26 2025 |
R-4.5-linux | OK | Mar 26 2025 |
R-4.4-linux | OK | Mar 26 2025 |
Exports:clear_labelscrrRxexcelColexcelColLettersextract_labelsforestplot2forestplotMVforestplotUVforestplotUVMVggkmcifggkmcif_pasteggkmcif2nestTableoutTableplotuvreplace_plot_labelsrm_cifsumrm_compactsumrm_covsumrm_mvsumrm_survdiffrm_survsumrm_survtimerm_uv_mvrm_uvsumscrolling_tableset_labelsset_var_labels
Dependencies:abindaodbackportsbase64encbootbroombslibcachemcarcarDataclicmprskcolorspacecorrplotcowplotcpp11DerivdigestdoBydplyrevaluatefansifarverfastmapfontawesomeFormulafsgeepackgenericsggplot2ggpubrggrepelggsciggsignifgluegridExtragtablehighrhtmltoolsisobandjquerylibjsonlitekableExtraknitrlabelinglatticelifecyclelme4magrittrMASSMatrixMatrixModelsmemoisemgcvmicrobenchmarkmimeminqamodelrmunsellnlmenloptrnnetnumDerivpanderpbkrtestpillarpkgconfigplyrpolynompurrrquantregR6rappdirsrbibutilsRColorBrewerRcppRcppEigenRdpackreformulasrlangrmarkdownrstatixrstudioapisassscalesSparseMstringistringrsurvivalsvglitesystemfontstibbletidyrtidyselecttinytexutf8vctrsviridisLitewithrxfunxml2yaml