Package: pcdpca 0.4
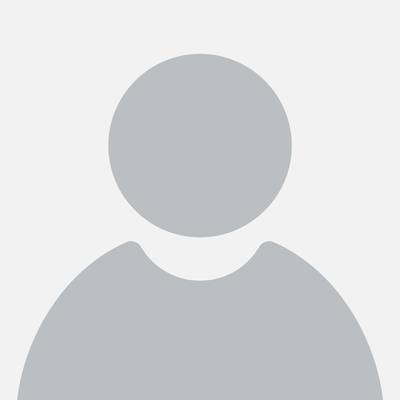
Lukasz Kidzinski
pcdpca: Dynamic Principal Components for Periodically Correlated Functional Time Series
Method extends multivariate and functional dynamic principal components to periodically correlated multivariate time series. This package allows you to compute true dynamic principal components in the presence of periodicity. We follow implementation guidelines as described in Kidzinski, Kokoszka and Jouzdani (2017), in Principal component analysis of periodically correlated functional time series <arxiv:1612.00040>.
Authors:
pcdpca_0.4.tar.gz
pcdpca_0.4.tar.gz(r-4.5-noble)pcdpca_0.4.tar.gz(r-4.4-noble)
pcdpca_0.4.tgz(r-4.4-emscripten)pcdpca_0.4.tgz(r-4.3-emscripten)
pcdpca.pdf |pcdpca.html✨
pcdpca/json (API)
# Install 'pcdpca' in R: |
install.packages('pcdpca', repos = c('https://cran.r-universe.dev', 'https://cloud.r-project.org')) |
This package does not link to any Github/Gitlab/R-forge repository. No issue tracker or development information is available.
Last updated 7 years agofrom:994e422255. Checks:OK: 1 NOTE: 1. Indexed: yes.
Target | Result | Date |
---|---|---|
Doc / Vignettes | OK | Aug 19 2024 |
R-4.5-linux | NOTE | Aug 19 2024 |
Exports:pcdpcapcdpca.inversepcdpca.scores
Dependencies:ashbitopscliclustercolorspacedeSolvefansifarverfdafdsFNNfreqdomggplot2gluegtablehdrcdeisobandkernlabKernSmoothkslabelinglatticelifecyclelocfitmagrittrMASSMatrixmatrixcalcmclustmgcvmulticoolmunsellmvtnormnlmepcaPPpillarpkgconfigpracmaR6rainbowRColorBrewerRcppRCurlrlangscalestibbleutf8vctrsviridisLitewithr
Readme and manuals
Help Manual
Help page | Topics |
---|---|
Compute periodically correlacted DPCA filter coefficients | pcdpca |
Retrieve a process from given scores | pcdpca.inverse |
Compute periodically correlated DPCA scores, given the filters XI | pcdpca.scores |