Package: lipidomeR 0.1.2
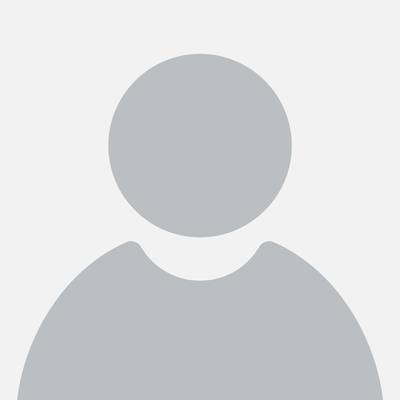
Tommi Suvitaival
lipidomeR: Integrative Visualizations of the Lipidome
Create lipidome-wide heatmaps of statistics with the 'lipidomeR'. The 'lipidomeR' provides a streamlined pipeline for the systematic interpretation of the lipidome through publication-ready visualizations of regression models fitted on lipidomics data. With 'lipidomeR', associations between covariates and the lipidome can be interpreted systematically and intuitively through heatmaps, where lipids are categorized by the lipid class and are presented on two-dimensional maps organized by the lipid size and level of saturation. This way, the 'lipidomeR' helps you gain an immediate understanding of the multivariate patterns in the lipidome already at first glance. You can create lipidome-wide heatmaps of statistical associations, changes, differences, variation, or other lipid-specific values. The heatmaps are provided with publication-ready quality and the results behind the visualizations are based on rigorous statistical models.
Authors:
lipidomeR_0.1.2.tar.gz
lipidomeR_0.1.2.tar.gz(r-4.5-noble)lipidomeR_0.1.2.tar.gz(r-4.4-noble)
lipidomeR_0.1.2.tgz(r-4.4-emscripten)lipidomeR_0.1.2.tgz(r-4.3-emscripten)
lipidomeR.pdf |lipidomeR.html✨
lipidomeR/json (API)
NEWS
# Install 'lipidomeR' in R: |
install.packages('lipidomeR', repos = c('https://cran.r-universe.dev', 'https://cloud.r-project.org')) |
- cancerlipidome - Levels of lipids in benign and malignant breast tumors in humans.
- humanlipidome - Concentrations of lipids in a human plasma reference sample
- liverlipidome - Levels of lipids in the human liver with or without non-alcoholic liver disease (NAFLD).
This package does not link to any Github/Gitlab/R-forge repository. No issue tracker or development information is available.
Last updated 5 years agofrom:cdaf354700. Checks:2 OK. Indexed: yes.
Target | Result | Latest binary |
---|---|---|
Doc / Vignettes | OK | Feb 24 2025 |
R-4.5-linux | OK | Feb 24 2025 |
Exports:compute_F_test_with_limmacompute_models_with_limmacompute_post_hoc_test_with_limmaheatmap_lipidomeheatmap_lipidome_from_limmainstall_Bioconductor_dependenciesmap_lipid_names
Dependencies:BiocManagerbitbit64classclicliprcolorspacecpp11crayonDBIdplyre1071evaluatefansifarverforcatsgdatagenericsggplot2gluegmodelsgtablegtoolshavenhighrhmsisobandknitrlabelinglabelledlatticelifecyclelimmamagrittrMASSMatrixmgcvminqamitoolsmunsellnlmenumDerivpillarpkgconfigplyrprettyunitsprogressproxypurrrR6RColorBrewerRcppRcppArmadilloreadrreshape2rlangscalesshadowtextstatmodstringistringrsurveysurvivaltableonetibbletidyrtidyselecttzdbutf8vctrsviridisLitevroomwithrxfunyamlzoo
Readme and manuals
Help Manual
Help page | Topics |
---|---|
Levels of lipids in benign and malignant breast tumors in humans. | cancerlipidome |
Compute an F-test for a multi-level factor independent variable | compute_F_test_with_limma |
Compute lipid-specific regression models | compute_models_with_limma |
Compute pairwise post-hoc comparisons for a multi-level factor | compute_post_hoc_test_with_limma |
Create 'lipidomeR' heatmaps of arbitrary lipid-specific values. | heatmap_lipidome |
Create 'lipidomeR' heatmaps of model statistics | heatmap_lipidome_from_limma |
Concentrations of lipids in a human plasma reference sample | humanlipidome |
Install the Bioconductor dependencies for the 'lipidomeR' | install_Bioconductor_dependencies |
Levels of lipids in the human liver with or without non-alcoholic liver disease (NAFLD). | liverlipidome |
Enumerate lipid names into values about lipid class, size and saturation | map_lipid_names |