Package: kko 1.0.1
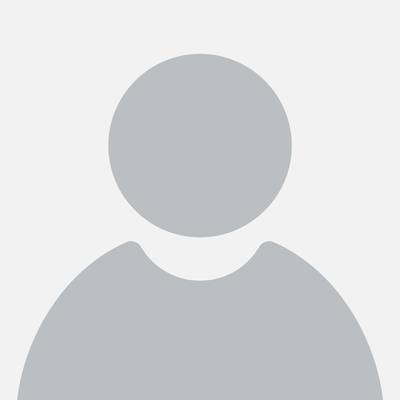
Xiang Lyu
kko: Kernel Knockoffs Selection for Nonparametric Additive Models
A variable selection procedure, dubbed KKO, for nonparametric additive model with finite-sample false discovery rate control guarantee. The method integrates three key components: knockoffs, subsampling for stability, and random feature mapping for nonparametric function approximation. For more information, see the accompanying paper: Dai, X., Lyu, X., & Li, L. (2021). “Kernel Knockoffs Selection for Nonparametric Additive Models”. arXiv preprint <arxiv:2105.11659>.
Authors:
kko_1.0.1.tar.gz
kko_1.0.1.tar.gz(r-4.5-noble)kko_1.0.1.tar.gz(r-4.4-noble)
kko_1.0.1.tgz(r-4.4-emscripten)kko_1.0.1.tgz(r-4.3-emscripten)
kko.pdf |kko.html✨
kko/json (API)
# Install 'kko' in R: |
install.packages('kko', repos = c('https://cran.r-universe.dev', 'https://cloud.r-project.org')) |
This package does not link to any Github/Gitlab/R-forge repository. No issue tracker or development information is available.
Last updated 3 years agofrom:de22258945. Checks:OK: 2. Indexed: yes.
Target | Result | Date |
---|---|---|
Doc / Vignettes | OK | Dec 01 2024 |
R-4.5-linux | OK | Dec 01 2024 |
Exports:generate_datakkoKO_evaluationrk_fitrk_subsamplerk_tune
Dependencies:codetoolscorpcordoParallelExtDistforeachglmnetgrpreggtoolsiteratorsknockofflatticeMatrixnloptrnumDerivoptimxpracmaRcppRcppEigenRdsdpRSpectrashapesurvival
Readme and manuals
Help Manual
Help page | Topics |
---|---|
generate response from nonparametric additive model | generate_data |
variable selection for additive model via KKO | kko |
evaluate performance of KKO selection | KO_evaluation |
nonparametric additive model seleciton via random kernel | rk_fit |
compute selection frequency of rk_fit on subsamples | rk_subsample |
tune random feature number for KKO. | rk_tune |