Package: irrCAC 1.0
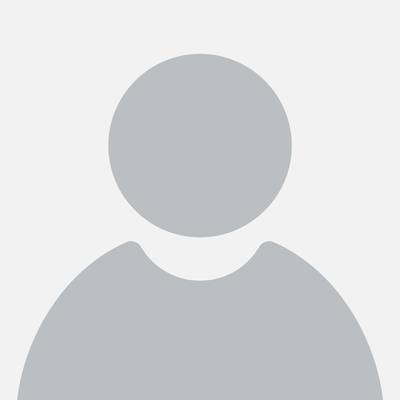
Kilem L. Gwet
irrCAC: Computing Chance-Corrected Agreement Coefficients (CAC)
Calculates various chance-corrected agreement coefficients (CAC) among 2 or more raters are provided. Among the CAC coefficients covered are Cohen's kappa, Conger's kappa, Fleiss' kappa, Brennan-Prediger coefficient, Gwet's AC1/AC2 coefficients, and Krippendorff's alpha. Multiple sets of weights are proposed for computing weighted analyses. All of these statistical procedures are described in details in Gwet, K.L. (2014,ISBN:978-0970806284): "Handbook of Inter-Rater Reliability," 4th edition, Advanced Analytics, LLC.
Authors:
irrCAC_1.0.tar.gz
irrCAC_1.0.tar.gz(r-4.5-noble)irrCAC_1.0.tar.gz(r-4.4-noble)
irrCAC_1.0.tgz(r-4.4-emscripten)irrCAC_1.0.tgz(r-4.3-emscripten)
irrCAC.pdf |irrCAC.html✨
irrCAC/json (API)
# Install 'irrCAC' in R: |
install.packages('irrCAC', repos = c('https://cran.r-universe.dev', 'https://cloud.r-project.org')) |
- altman - Dataset describing the Altman's Benchmarking Scale
- cac.ben.gerry - Ratings of 12 units from 2 raters named Ben and Gerry
- cac.dist.g1g2 - Distribution of 4 raters by subject and by category, for 14 Subjects that belong to 2 groups "G1" and "G2"
- cac.dist4cat - Distribution of 4 raters by Category and Subject - Subjects allocated in 2 groups A and B.
- cac.raw.g1g2 - Dataset of raw ratings from 4 Raters on 14 Subjects that belong to 2 groups named "G1" and "G2"
- cac.raw.gender - Rating Data from 4 Raters and 15 human Subjects, 9 of whom are female and 6 males.
- cac.raw4raters - Rating Data from 4 Raters and 12 Subjects.
- cac.raw5obser - Scores assigned by 5 observers to 20 experimental units.
- cont3x3abstractors - Distribution of 100 pregnant women by pregnancy type and by abstractor.
- cont4x4diagnosis - Distribution of 223 Psychiatric Patients by Type of of Psychiatric Disorder and Diagnosis Method.
- distrib.6raters - Distribution of 6 psychiatrists by Subject/patient and diagnosis Category.
- fleiss - Dataset describing Fleiss' Benchmarking Scale
- landis.koch - Dataset describing the Landis & Koch Benchmarking Scale
This package does not link to any Github/Gitlab/R-forge repository. No issue tracker or development information is available.
Last updated 5 years agofrom:41b40b431b. Checks:1 OK, 1 NOTE. Indexed: yes.
Target | Result | Latest binary |
---|---|---|
Doc / Vignettes | OK | Feb 08 2025 |
R-4.5-linux | NOTE | Feb 08 2025 |
Exports:altman.bfbipolar.weightsbp.coeff.distbp.coeff.rawbp2.tablecircular.weightsconger.kappa.rawfleiss.bffleiss.kappa.distfleiss.kappa.rawgwet.ac1.distgwet.ac1.rawgwet.ac1.tableidentity.weightskappa2.tablekrippen.alpha.distkrippen.alpha.rawkrippen2.tablelandis.koch.bflinear.weightsordinal.weightspa.coeff.distpa.coeff.rawpa2.tablequadratic.weightsradical.weightsratio.weightsscott2.table
Dependencies:
irrCAC-benchmarking
Rendered frombenchmarking.Rmd
usingknitr::rmarkdown
on Feb 08 2025.Last update: 2019-09-23
Started: 2019-09-23
Calculating Chance-corrected Agreement Coefficients (CAC)
Rendered fromoverview.Rmd
usingknitr::rmarkdown
on Feb 08 2025.Last update: 2019-09-23
Started: 2019-09-23
Weighted Chance-corrected Agreement Coefficients
Rendered fromweighting.Rmd
usingknitr::rmarkdown
on Feb 08 2025.Last update: 2019-09-23
Started: 2019-09-23