Package: inaparc 1.2.0
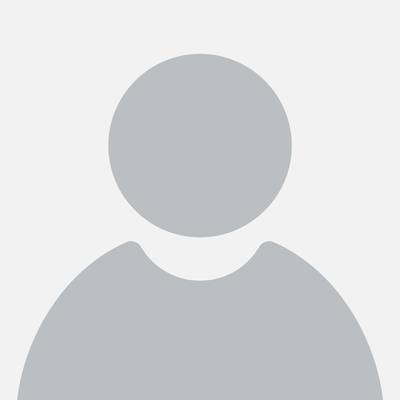
Zeynel Cebeci
inaparc: Initialization Algorithms for Partitioning Cluster Analysis
Partitioning clustering algorithms divide data sets into k subsets or partitions so-called clusters. They require some initialization procedures for starting the algorithms. Initialization of cluster prototypes is one of such kind of procedures for most of the partitioning algorithms. Cluster prototypes are the centers of clusters, i.e. centroids or medoids, representing the clusters in a data set. In order to initialize cluster prototypes, the package 'inaparc' contains a set of the functions that are the implementations of several linear time-complexity and loglinear time-complexity methods in addition to some novel techniques. Initialization of fuzzy membership degrees matrices is another important task for starting the probabilistic and possibilistic partitioning algorithms. In order to initialize membership degrees matrices required by these algorithms, a number of functions based on some traditional and novel initialization techniques are also available in the package 'inaparc'.
Authors:
inaparc_1.2.0.tar.gz
inaparc_1.2.0.tar.gz(r-4.5-noble)inaparc_1.2.0.tar.gz(r-4.4-noble)
inaparc_1.2.0.tgz(r-4.4-emscripten)inaparc_1.2.0.tgz(r-4.3-emscripten)
inaparc.pdf |inaparc.html✨
inaparc/json (API)
NEWS
# Install 'inaparc' in R: |
install.packages('inaparc', repos = c('https://cran.r-universe.dev', 'https://cloud.r-project.org')) |
This package does not link to any Github/Gitlab/R-forge repository. No issue tracker or development information is available.
Last updated 2 years agofrom:cf23549379. Checks:OK: 1 NOTE: 1. Indexed: yes.
Target | Result | Date |
---|---|---|
Doc / Vignettes | OK | Aug 25 2024 |
R-4.5-linux | NOTE | Aug 25 2024 |
Exports:aldaoudballhallcrsampfigenfirstkforgyget.algorithmshartiganwongimembonesimembrandinofrepinscsfinsdevis.inaparckkzkmppksegmentskstepslastklhsmaximinlhsrandommaximinmscseekrsamprsegmentscseekscseek2spaethssamptopbottomuniquekursamp