Package: changepoints 1.1.0
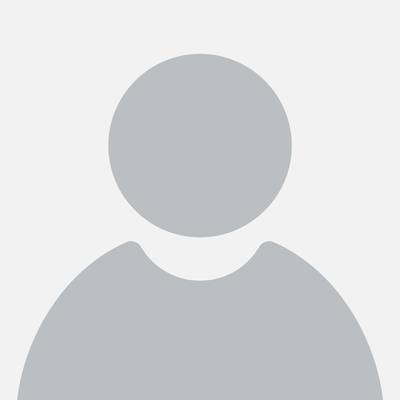
Haotian Xu
changepoints: A Collection of Change-Point Detection Methods
Performs a series of offline and/or online change-point detection algorithms for 1) univariate mean: <doi:10.1214/20-EJS1710>, <arxiv:2006.03283>; 2) univariate polynomials: <doi:10.1214/21-EJS1963>; 3) univariate and multivariate nonparametric settings: <doi:10.1214/21-EJS1809>, <doi:10.1109/TIT.2021.3130330>; 4) high-dimensional covariances: <doi:10.3150/20-BEJ1249>; 5) high-dimensional networks with and without missing values: <doi:10.1214/20-AOS1953>, <arxiv:2101.05477>, <arxiv:2110.06450>; 6) high-dimensional linear regression models: <arxiv:2010.10410>, <arxiv:2207.12453>; 7) high-dimensional vector autoregressive models: <arxiv:1909.06359>; 8) high-dimensional self exciting point processes: <arxiv:2006.03572>; 9) dependent dynamic nonparametric random dot product graphs: <arxiv:1911.07494>; 10) univariate mean against adversarial attacks: <arxiv:2105.10417>.
Authors:
changepoints_1.1.0.tar.gz
changepoints_1.1.0.tar.gz(r-4.5-noble)changepoints_1.1.0.tar.gz(r-4.4-noble)
changepoints_1.1.0.tgz(r-4.4-emscripten)changepoints_1.1.0.tgz(r-4.3-emscripten)
changepoints.pdf |changepoints.html✨
changepoints/json (API)
NEWS
# Install 'changepoints' in R: |
install.packages('changepoints', repos = c('https://cran.r-universe.dev', 'https://cloud.r-project.org')) |
Bug tracker:https://github.com/haotianxu/changepoints/issues
Last updated 2 years agofrom:2dd2969c28. Checks:1 OK, 1 NOTE. Indexed: no.
Target | Result | Latest binary |
---|---|---|
Doc / Vignettes | OK | Jan 06 2025 |
R-4.5-linux-x86_64 | NOTE | Jan 06 2025 |
Exports:aARCARCBD_UBS.covBS.uni.nonparBS.univarcalibrate.online.network.missingCI.regressionCV.search.DP.LR.regressionCV.search.DP.polyCV.search.DP.regressionCV.search.DP.univarCV.search.DP.VAR1CV.search.DPDU.regressionDP.polyDP.regressionDP.SEPPDP.univarDP.VAR1DPDU.regressiongen.cov.matgen.missinggen.piece.polygen.piece.poly.noiselessHausdorff.disthuber_meanlambda.network.missinglocal.refine.CV.VAR1local.refine.DPDU.regressionlocal.refine.networklocal.refine.polylocal.refine.regressionlocal.refine.univarlocal.refine.VAR1lowertri2matLRV.regressiononline.networkonline.network.missingonline.univaronline.univar.multisimu.change.regressionsimu.RDPGsimu.SBMsimu.SEPPsimu.VAR1softImpute.network.missingthresholdBStrim_intervaltuneBSmultinonpartuneBSnonparRDPGtuneBSuninonpartuneBSunivarWBS.intervalsWBS.multi.nonparWBS.networkWBS.nonpar.RDPGWBS.uni.nonparWBS.uni.robWBS.univarWBSIP.cov
Dependencies:codetoolsdata.treeFNNforeachgglassoglmnetiteratorskernlabKernSmoothkslatticeMASSMatrixmclustmgcvmulticoolmvtnormnlmepracmaR6RcppRcppArmadilloRcppEigenshapestringisurvival