Package: TransTGGM 1.0.0
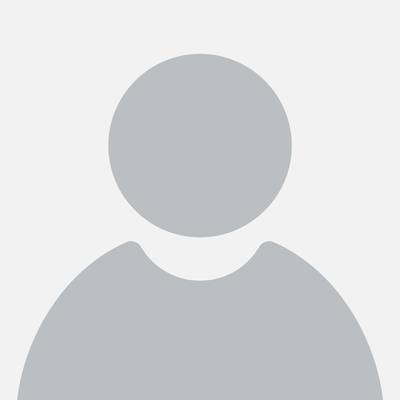
Mingyang Ren
TransTGGM: Transfer Learning for Tensor Graphical Models
Tensor Gaussian graphical models (GGMs) have important applications in numerous areas, which can interpret conditional independence structures within tensor data. Yet, the available tensor data in one single study is often limited due to high acquisition costs. Although relevant studies can provide additional data, it remains an open question how to pool such heterogeneous data. This package implements a transfer learning framework for tensor GGMs, which takes full advantage of informative auxiliary domains even when non-informative auxiliary domains are present, benefiting from the carefully designed data-adaptive weights. Reference: Ren, M., Zhen Y., and Wang J. (2022). "Transfer learning for tensor graphical models" <arxiv:2211.09391>.
Authors:
TransTGGM_1.0.0.tar.gz
TransTGGM_1.0.0.tar.gz(r-4.5-noble)TransTGGM_1.0.0.tar.gz(r-4.4-noble)
TransTGGM_1.0.0.tgz(r-4.4-emscripten)TransTGGM_1.0.0.tgz(r-4.3-emscripten)
TransTGGM.pdf |TransTGGM.html✨
TransTGGM/json (API)
# Install 'TransTGGM' in R: |
install.packages('TransTGGM', repos = c('https://cran.r-universe.dev', 'https://cloud.r-project.org')) |
- example.data - Some example data
This package does not link to any Github/Gitlab/R-forge repository. No issue tracker or development information is available.
Last updated 2 years agofrom:19e4ac8aac. Checks:OK: 1 NOTE: 1. Indexed: yes.
Target | Result | Date |
---|---|---|
Doc / Vignettes | OK | Dec 06 2024 |
R-4.5-linux | NOTE | Dec 06 2024 |
Exports:tensor.GGM.transTheta.estTheta.tuning
Dependencies:clicodetoolscpp11doParallelexpmforeachglassogluehugeigraphiteratorslatticelifecyclemagrittrMASSMatrixpkgconfigRcppRcppEigenrlangrTensorTlassovctrs
Readme and manuals
Help Manual
Help page | Topics |
---|---|
Some example data | example.data |
Transfer learning for tensor graphical models. | tensor.GGM.trans |
Fast sparse precision matrix estimation. | Theta.est |
Fast sparse precision matrix estimation. | Theta.tuning |