Package: SpatialML 0.1.7
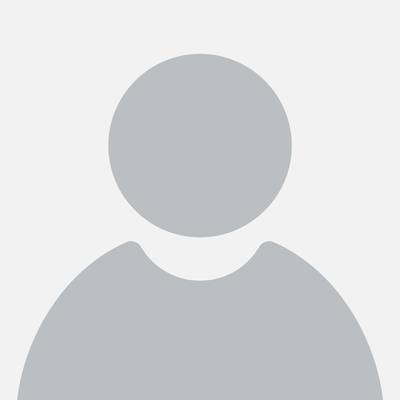
Stamatis Kalogirou
SpatialML: Spatial Machine Learning
Implements a spatial extension of the random forest algorithm (Georganos et al. (2019) <doi:10.1080/10106049.2019.1595177>). Allows for a geographically weighted random forest regression including a function to find the optical bandwidth. (Georganos and Kalogirou (2022) <https://www.mdpi.com/2220-9964/11/9/471>).
Authors:
SpatialML_0.1.7.tar.gz
SpatialML_0.1.7.tar.gz(r-4.5-noble)SpatialML_0.1.7.tar.gz(r-4.4-noble)
SpatialML_0.1.7.tgz(r-4.4-emscripten)SpatialML_0.1.7.tgz(r-4.3-emscripten)
SpatialML.pdf |SpatialML.html✨
SpatialML/json (API)
# Install 'SpatialML' in R: |
install.packages('SpatialML', repos = c('https://cran.r-universe.dev', 'https://cloud.r-project.org')) |
- Income - Mean household income at lcoal authorities in Greece in 2011
This package does not link to any Github/Gitlab/R-forge repository. No issue tracker or development information is available.
Last updated 9 months agofrom:621a1dc467. Checks:OK: 1 NOTE: 1. Indexed: yes.
Target | Result | Date |
---|---|---|
Doc / Vignettes | OK | Nov 28 2024 |
R-4.5-linux | NOTE | Nov 28 2024 |
Exports:grfgrf.bwpredict.grfrandom.test.datarf.mtry.optim
Dependencies:caretclasscliclockcodetoolscolorspacecpp11data.tablediagramdigestdplyre1071fansifarverforeachfuturefuture.applygenericsggplot2globalsgluegowergtablehardhatipredisobanditeratorsKernSmoothlabelinglatticelavalifecyclelistenvlubridatemagrittrMASSMatrixmgcvModelMetricsmunsellnlmennetnumDerivparallellypillarpkgconfigplyrpROCprodlimprogressrproxypurrrR6randomForestrangerRColorBrewerRcppRcppEigenrecipesreshape2rlangrpartscalesshapeSQUAREMstringistringrsurvivaltibbletidyrtidyselecttimechangetimeDatetzdbutf8vctrsviridisLitewithr
Readme and manuals
Help Manual
Help page | Topics |
---|---|
Geographically Weighted Random Forest Model | grf |
Geographically Weighted Random Forest optimal bandwidth selection | grf.bw |
Mean household income at lcoal authorities in Greece in 2011 | Income |
Predict Method for Geographical Random Forest | predict.grf |
Radmom data generator | random.test.data |
Optimal mtry | rf.mtry.optim |