Package: SILGGM 1.0.0
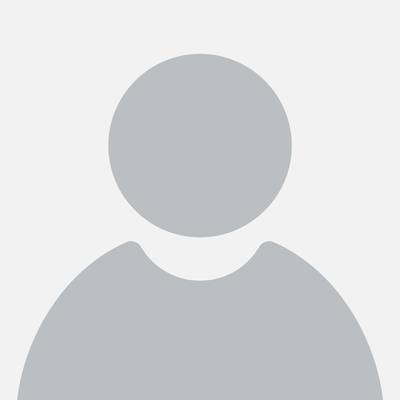
Rong Zhang
SILGGM: Statistical Inference of Large-Scale Gaussian Graphical Model in Gene Networks
Provides a general framework to perform statistical inference of each gene pair and global inference of whole-scale gene pairs in gene networks using the well known Gaussian graphical model (GGM) in a time-efficient manner. We focus on the high-dimensional settings where p (the number of genes) is allowed to be far larger than n (the number of subjects). Four main approaches are supported in this package: (1) the bivariate nodewise scaled Lasso (Ren et al (2015) <doi:10.1214/14-AOS1286>) (2) the de-sparsified nodewise scaled Lasso (Jankova and van de Geer (2017) <doi:10.1007/s11749-016-0503-5>) (3) the de-sparsified graphical Lasso (Jankova and van de Geer (2015) <doi:10.1214/15-EJS1031>) (4) the GGM estimation with false discovery rate control (FDR) using scaled Lasso or Lasso (Liu (2013) <doi:10.1214/13-AOS1169>). Windows users should install 'Rtools' before the installation of this package.
Authors:
SILGGM_1.0.0.tar.gz
SILGGM_1.0.0.tar.gz(r-4.5-noble)SILGGM_1.0.0.tar.gz(r-4.4-noble)
SILGGM_1.0.0.tgz(r-4.4-emscripten)SILGGM_1.0.0.tgz(r-4.3-emscripten)
SILGGM.pdf |SILGGM.html✨
SILGGM/json (API)
# Install 'SILGGM' in R: |
install.packages('SILGGM', repos = c('https://cran.r-universe.dev', 'https://cloud.r-project.org')) |
This package does not link to any Github/Gitlab/R-forge repository. No issue tracker or development information is available.
Last updated 7 years agofrom:1b00328765. Checks:OK: 2. Indexed: yes.
Target | Result | Date |
---|---|---|
Doc / Vignettes | OK | Aug 30 2024 |
R-4.5-linux-x86_64 | OK | Aug 30 2024 |
Exports:SILGGM