Package: RclusTool 0.91.6
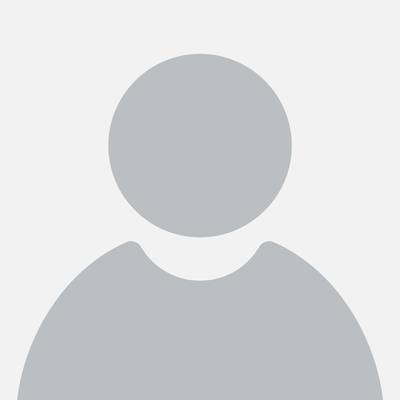
Pierre-Alexandre Hebert
RclusTool: Graphical Toolbox for Clustering and Classification of Data Frames
Graphical toolbox for clustering and classification of data frames. It proposes a graphical interface to process clustering and classification methods on features data-frames, and to view initial data as well as resulted cluster or classes. According to the level of available labels, different approaches are proposed: unsupervised clustering, semi-supervised clustering and supervised classification. To assess the processed clusters or classes, the toolbox can import and show some supplementary data formats: either profile/time series, or images. These added information can help the expert to label clusters (clustering), or to constrain data frame rows (semi-supervised clustering), using Constrained spectral embedding algorithm by Wacquet et al. (2013) <doi:10.1016/j.patrec.2013.02.003> and the methodology provided by Wacquet et al. (2013) <doi:10.1007/978-3-642-35638-4_21>.
Authors:
RclusTool_0.91.6.tar.gz
RclusTool_0.91.6.tar.gz(r-4.5-noble)RclusTool_0.91.6.tar.gz(r-4.4-noble)
RclusTool_0.91.6.tgz(r-4.4-emscripten)RclusTool_0.91.6.tgz(r-4.3-emscripten)
RclusTool.pdf |RclusTool.html✨
RclusTool/json (API)
# Install 'RclusTool' in R: |
install.packages('RclusTool', repos = c('https://cran.r-universe.dev', 'https://cloud.r-project.org')) |
This package does not link to any Github/Gitlab/R-forge repository. No issue tracker or development information is available.
Last updated 10 months agofrom:1163374f5c. Checks:OK: 2. Indexed: yes.
Target | Result | Date |
---|---|---|
Doc / Vignettes | OK | Dec 24 2024 |
R-4.5-linux | OK | Dec 24 2024 |
Exports:abdPlotabdPlotTabsabdPlotTabsGUIaddClusteringaddIds2SamplingaddOperationanalyzePlotapplyPreprocessingbipartitionShibuildBatchTabbuildClusteringSamplebuildConstraintsMatrixbuildImportTabbuildNameOperationbuildPreprocessTabbuildSemisupTabbuildsupTabbuildUnsupTabclusterDensityclusterSummarycomputeCKmeanscomputeCSCcomputeEMcomputeGapcomputeGap2computeGaussianSimilaritycomputeGaussianSimilarityZPcomputeItemsSamplecomputeItemsSampleGUIcomputeKmeanscomputePcaNbDimscomputePcaSamplecomputeSamplingcomputeSemiSupervisedcomputeSpectralEmbeddingSamplecomputeSupervisedcomputeUnSupervisedconvNamesPairsToIndexPairsconvNamesToIndexcor.mtestcountItemscountItemsSampleGUIcreateResFoldercritMNCutdetailOperationdropTrainSetVarsElbowFinderElbowPlotextractFeaturesFromSummaryextractProtosfeatSpaceNameConvertFindNumberKformatLabelSampleformatParameterListguessFileEncodingimgClassifimportLabelSampleimportSampleinitBatchTabinitImportTabinitParametersinitPreprocessTabinitSemisupTabinitSupTabinitUnsupTabitemsModelKmeansAutoElbowKmeansQuickKwaySSSClistDerivableFeatureSpacesloadPreprocessFileloadPreviousResloadSampleloadSummaryMainWindowmakeFeatureSpaceOperationsmakeTitlematchNamesmeasureConstraintsOkmeasureMNCutmessageConsolenameClustersplotDensity2DplotProfileplotProfileExtractplotSampleFeaturespreviewCSVfilepurgeSampleRclusToolGUIreadTrainSetremoveZerossaveCalculsaveClusteringsaveCountssaveLogFilesaveManualProtossavePreprocesssaveSummarysearch.neighboursigClassifsortCharAsNumsortLabelspectralClusteringspectralClusteringNgspectralEmbeddingNgtk2add.notetabtk2delete.notetabtk2draw.notetabtk2notetab.RclusTooltkEmptyLinetkrplot.RclusTooltkrreplot.RclusTooltoStringDataFrameupdateClustersNamesvisualizeSampleClustering
Dependencies:abindbackportsbase64encbootbroombslibcachemcarcarDataclasscliclustercolorspaceconclustcorrplotcowplotcpp11crosstalkdendextendDerivdigestdoBydplyrDTe1071ellipseemmeansestimabilityevaluatefactoextraFactoMineRfansifarverfastmapflashClustfontawesomeFormulafsgenericsggplot2ggpubrggrepelggsciggsignifgluegridExtragtablehighrhtmltoolshtmlwidgetshttpuvisobandjpegjquerylibjsonliteknitrlabelinglaterlatticelazyevalleapslifecyclelme4magrittrMASSMatrixMatrixModelsmclustmdamemoisemgcvmicrobenchmarkmimeminqammandmodelrmultcompViewmunsellmvtnormnlmenloptrnnetnumDerivpbkrtestpillarpkgconfigplyrpngpolynompromisesproxypurrrquantregR6randomForestrappdirsRColorBrewerRcppRcppEigenreshapereshape2rlangrmarkdownrstatixsassscalesscatterplot3dSearchTreesspSparseMstringistringrsurvivaltcltk2tibbletidyrtidyselecttinytextkrplotutf8vctrsviridisviridisLitewithrxfunyaml
Readme and manuals
Help Manual
Help page | Topics |
---|---|
Add operation | addOperation |
Preprocessing application | applyPreprocessing |
Clusters summaries computation | clusterSummary |
Semi-supervised clustering | computeSemiSupervised |
Supervised classification | computeSupervised |
Unsupervised clustering | computeUnSupervised |
Prototypes extraction | extractProtos |
Labels formatting | formatLabelSample |
Images clustering | imgClassif |
Sample importation | importSample |
Preprocessing loading | loadPreprocessFile |
Sample purging | purgeSample |
Username and user type selection | RclusToolGUI |
Training set reading | readTrainSet |
Object saving | saveCalcul |
Clustering saving | saveClustering |
Count saving | saveCounts |
Manual prototypes saving | saveManualProtos |
Preprocessing exportation | savePreprocess |
Clusters summaries saving | saveSummary |
Signals clustering | sigClassif |
Interactive figure with 2D scatter-plot | visualizeSampleClustering |