Package: MLCOPULA 1.0.1
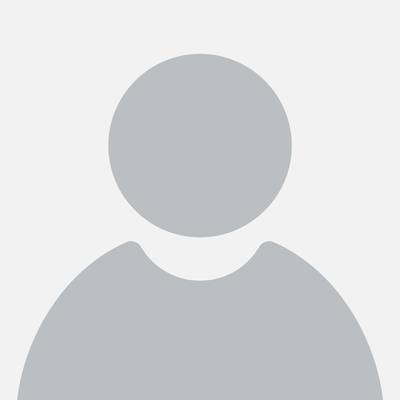
Rogelio Salinas Gutiérrez
MLCOPULA: Classification Models with Copula Functions
Provides several classifiers based on probabilistic models. These classifiers allow to model the dependence structure of continuous features through bivariate copula functions and graphical models, see Salinas-Gutiérrez et al. (2014) <doi:10.1007/s00180-013-0457-y>.
Authors:
MLCOPULA_1.0.1.tar.gz
MLCOPULA_1.0.1.tar.gz(r-4.5-noble)MLCOPULA_1.0.1.tar.gz(r-4.4-noble)
MLCOPULA_1.0.1.tgz(r-4.4-emscripten)MLCOPULA_1.0.1.tgz(r-4.3-emscripten)
MLCOPULA.pdf |MLCOPULA.html✨
MLCOPULA/json (API)
# Install 'MLCOPULA' in R: |
install.packages('MLCOPULA', repos = 'https://cloud.r-project.org') |
This package does not link to any Github/Gitlab/R-forge repository. No issue tracker or development information is available.
Last updated 5 months agofrom:94b868dd9b. Checks:3 OK. Indexed: yes.
Target | Result | Latest binary |
---|---|---|
Doc / Vignettes | OK | Mar 23 2025 |
R-4.5-linux | OK | Mar 23 2025 |
R-4.4-linux | OK | Mar 23 2025 |
Exports:classification_reportcopulaClassifiercopulaPredict
Dependencies:ADGofTestBHclicodetoolscolorspacecopulacpp11dotCall64evmixfansifarverfieldsforeachggplot2glueGRIDCOPULAgslgtableigraphisobanditeratorskde1dlabelinglatticelifecyclelimSolvelpSolvemagrittrmapsMASSMatrixmgcvmunsellmvtnormnlmenumDerivpcaPPpillarpkgconfigplyrpracmapsplinequadprogR6randtoolboxRColorBrewerRcppRcppEigenreshape2rlangrngWELLRsolnpscalesspamSparseMstablediststringistringrtibbletruncnormTSPutf8vctrsviridisLitewithr
Citation
To cite package ‘MLCOPULA’ in publications use:
Salinas Gutiérrez R, Hernández Quintero A, Montoya Calzada P, López Hernández C, Marquez Romero J (2024). MLCOPULA: Classification Models with Copula Functions. R package version 1.0.1, https://CRAN.R-project.org/package=MLCOPULA.
Corresponding BibTeX entry:
@Manual{, title = {MLCOPULA: Classification Models with Copula Functions}, author = {Rogelio {Salinas Gutiérrez} and Angélica {Hernández Quintero} and Pedro Abraham {Montoya Calzada} and Carlos Alberto {López Hernández} and Juan Manuel {Marquez Romero}}, year = {2024}, note = {R package version 1.0.1}, url = {https://CRAN.R-project.org/package=MLCOPULA}, }
Readme and manuals
MLCOPULA
This package implements 7 copulas for supervised classification: frank, gaussian, clayton, joe, gumbel, AMH and grid. The classification model is based on the Bayes theorem, similar to the naive Bayes classifier model, but does not assume that the features are independent.
The probability of a class given a set of characteristics (predictor variables) is:
$$P(A|x_1,..x_d) \alpha \prod_{i = 1}^{d}f_{X_i|A}(x_i)c(u_1,...,u_i)$$
where each $u_i = F_{X_i|A}(x_i)$ with $i = 1,2,..d$.
The copula density function $c(u_1,..u_i)$ is modeled by bivariate copula functions, using graphical models (trees and chains)
Copulas
For more details and visualization of the copulas, go to https://abrahammontoyacalzada.shinyapps.io/Copulas/
Frank copula:
$$C(u_1,u_2;\theta) = -\frac{1}{\theta} ln \left[ 1 + \frac{(e^{-\theta u_1} - 1) (e^{-\theta u_2} - 1) } {e^{-\theta} - 1} \right]$$
with $\theta \in (-\infty,\infty)/0$
This copula has no upper nor lower tail dependency.
Clayton copula:
$$C(u_1,u_2;\theta) = \left( u_1^{-\theta} + u_2^{-\theta} - 1 \right)^{-1/\theta}$$
with $\theta \in [-1,\infty)/0$
When $\theta \geq 0$ has lower tail dependence equal to $\lambda_L = 2^{-1/\theta}$
Gaussiana (Normal) copula $$C(u_1,u_2;\theta) = \Phi_G (\Phi^{-1} (u_1) , \Phi^{-1} (u_2) )$$
with $\theta \in (-1,1)$
This copula has no upper nor lower tail dependency.
Joe copula $$C(u_1,u_2) = 1 - \left[ (1 - u_1)^\theta + (1 - u_2)^\theta - (1 - u_1)^\theta (1 - u_2)^\theta \right ] ^ {1/\theta}$$
with $\theta \in [1,\infty)$
This copula has upper tail dependence equal to $\lambda_U = 2 - 2^{1/\theta}$
Gumbel copula
$$C(u_1,u_2) = exp \left[ - \left[ ( -ln(u_1) )^\theta + ( -ln(u_2) )^\theta \right]^{1/\theta} \right]$$
with $\theta \in [1,\infty)$
This copula has upper tail dependence equal to $\lambda_U = 2 - 2^{1/\theta}$
Ali–Mikhail–Haq copula
$$C(u_1,u_2) = \frac{u_1 u_2}{1 - \theta (1 - u_1)(1- u_2)}$$
with $\theta \in [-1,1)$
This copula has no upper nor lower tail dependency.
Example for iris data set
library(MLCOPULA)
X <- iris[,1:4]
y <- iris$Species
model <- copulaClassifier(X = X, y = y, copula = "frank",
distribution = "kernel", graph_model = "tree")
y_pred <- copulaPredict(X = X, model = model)
table(y,y_pred$class)
#Example 2
X <- iris[,1:4]
y <- iris$Species
model <- copulaClassifier(X = X, y = y, copula = c("frank","clayton"),
distribution = "kernel", graph_model = "chain")
y_pred <- copulaPredict(X = X, model = model)
table(y,y_pred$class)
References
Salinas-Gutiérrez, R., Hernández-Aguirre, A., Villa-Diharce, E.R. (2014). Copula selection for graphical models in continuous Estimation of Distribution Algorithms. Computational Statistics, 29(3–4):685–713. https://doi.org/10.1007/s00180-013-0457-y
Help Manual
Help page | Topics |
---|---|
Calculates classification performance metrics. | classification_report |
Trains a classification model using copula functions. | copulaClassifier |
Gets predictions from a classification model. | copulaPredict |