Package: EDISON 1.1.1
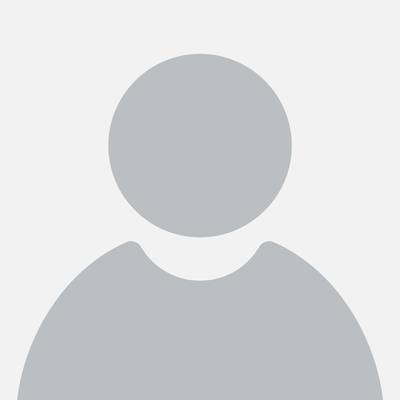
Frank Dondelinger
EDISON: Network Reconstruction and Changepoint Detection
Package EDISON (Estimation of Directed Interactions from Sequences Of Non-homogeneous gene expression) runs an MCMC simulation to reconstruct networks from time series data, using a non-homogeneous, time-varying dynamic Bayesian network. Networks segments and changepoints are inferred concurrently, and information sharing priors provide a reduction of the inference uncertainty.
Authors:
EDISON_1.1.1.tar.gz
EDISON_1.1.1.tar.gz(r-4.5-noble)EDISON_1.1.1.tar.gz(r-4.4-noble)
EDISON_1.1.1.tgz(r-4.4-emscripten)EDISON_1.1.1.tgz(r-4.3-emscripten)
EDISON.pdf |EDISON.html✨
EDISON/json (API)
NEWS
# Install 'EDISON' in R: |
install.packages('EDISON', repos = c('https://cran.r-universe.dev', 'https://cloud.r-project.org')) |
This package does not link to any Github/Gitlab/R-forge repository. No issue tracker or development information is available.
Last updated 9 years agofrom:9d3d366062. Checks:2 OK. Indexed: yes.
Target | Result | Latest binary |
---|---|---|
Doc / Vignettes | OK | Feb 08 2025 |
R-4.5-linux | OK | Feb 08 2025 |
Exports:AcceptableMoveaddProposalNetworkInfoBinoHyperMoveBinoHyperRatiobp.computeAlphabuildXYCalculateChangescalculateCPPGlobalcalculateCPProbabilitiescalculateEdgeProbabilitiescalculateEdgeProbabilitiesSegscalculateEdgeProbabilitiesTimePointsCalculateLikelihoodRatioCalculatePriorRatioCollectNetworkInfocomputePxcomputeRho4convert_netscp.birthcp.deathcp.shiftdefaultOptionsdinvgammaEDISON.runExpHyperMoveExpHyperRatioTargetfix_eigenvaluesgenerateNetworkHyperparameterMoveHyperParmsinitmainmake_structure_moveNetworkProbBinoNetworkProbExpNetworkRatioBinoNetworkRatioExpoutputphase.updatePriorRatioPoissonproposalTuningproposeContinuousProposeDiscretepsrfpsrf_checkpsrf_check_hyperreadDataTSrinvgammarunDBNsampleBinitsampleBxysampleDelta2sampleKsampleParmssampleSig2simulateNetworkupdateSigMultiupdateSigSolo