Package: EBglmnet 6.0
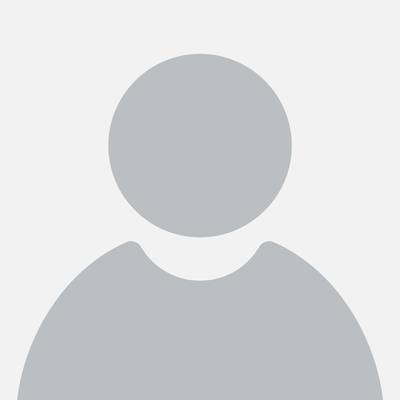
Anhui Huang
EBglmnet: Empirical Bayesian Lasso and Elastic Net Methods for Generalized Linear Models
Provides empirical Bayesian lasso and elastic net algorithms for variable selection and effect estimation. Key features include sparse variable selection and effect estimation via generalized linear regression models, high dimensionality with p>>n, and significance test for nonzero effects. This package outperforms other popular methods such as lasso and elastic net methods in terms of power of detection, false discovery rate, and power of detecting grouping effects. Please reference its use as A Huang and D Liu (2016) <doi:10.1093/bioinformatics/btw143>.
Authors:
EBglmnet_6.0.tar.gz
EBglmnet_6.0.tar.gz(r-4.5-noble)EBglmnet_6.0.tar.gz(r-4.4-noble)
EBglmnet_6.0.tgz(r-4.4-emscripten)EBglmnet_6.0.tgz(r-4.3-emscripten)
EBglmnet.pdf |EBglmnet.html✨
EBglmnet/json (API)
# Install 'EBglmnet' in R: |
install.packages('EBglmnet', repos = c('https://cran.r-universe.dev', 'https://cloud.r-project.org')) |
- BASIS - An Example Data
This package does not link to any Github/Gitlab/R-forge repository. No issue tracker or development information is available.
Last updated 1 years agofrom:f4c2448d0b. Checks:OK: 1 WARNING: 1. Indexed: yes.
Target | Result | Date |
---|---|---|
Doc / Vignettes | OK | Nov 01 2024 |
R-4.5-linux-x86_64 | WARNING | Nov 01 2024 |
Exports:cv.EBglmnetCVonePairEBelasticNet.BinomialEBelasticNet.BinomialCVEBelasticNet.GaussianEBelasticNet.GaussianCVEBglmnetEBlassoNE.BinomialCVEBlassoNE.GaussianCVEBlassoNEG.BinomialEBlassoNEG.BinomialCVEBlassoNEG.GaussianEBlassoNEG.GaussianCVijIndexlambdaMax
Dependencies: