Package: ARMALSTM 0.1.0
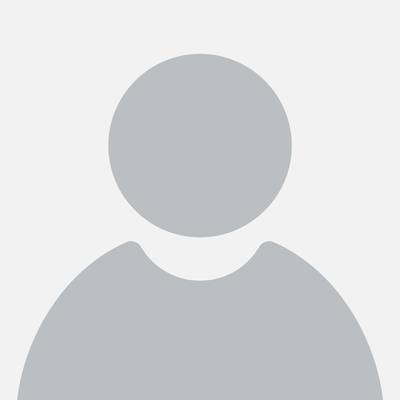
Debopam Rakshit
ARMALSTM: Fitting of Hybrid ARMA-LSTM Models
The real-life time series data are hardly pure linear or nonlinear. Merging a linear time series model like the autoregressive moving average (ARMA) model with a nonlinear neural network model such as the Long Short-Term Memory (LSTM) model can be used as a hybrid model for more accurate modeling purposes. Both the autoregressive integrated moving average (ARIMA) and autoregressive fractionally integrated moving average (ARFIMA) models can be implemented. Details can be found in Box et al. (2015, ISBN: 978-1-118-67502-1) and Hochreiter and Schmidhuber (1997) <doi:10.1162/neco.1997.9.8.1735>.
Authors:
ARMALSTM_0.1.0.tar.gz
ARMALSTM_0.1.0.tar.gz(r-4.5-noble)ARMALSTM_0.1.0.tar.gz(r-4.4-noble)
ARMALSTM_0.1.0.tgz(r-4.4-emscripten)ARMALSTM_0.1.0.tgz(r-4.3-emscripten)
ARMALSTM.pdf |ARMALSTM.html✨
ARMALSTM/json (API)
# Install 'ARMALSTM' in R: |
install.packages('ARMALSTM', repos = c('https://cran.r-universe.dev', 'https://cloud.r-project.org')) |
This package does not link to any Github/Gitlab/R-forge repository. No issue tracker or development information is available.
Last updated 1 years agofrom:050c704c2c. Checks:3 OK. Indexed: yes.
Target | Result | Latest binary |
---|---|---|
Doc / Vignettes | OK | Mar 25 2025 |
R-4.5-linux | OK | Mar 25 2025 |
R-4.4-linux | OK | Mar 25 2025 |
Exports:ARMA.LSTM
Dependencies:backportsbase64encchroncliconfigcurlDistributionUtilsFNNfracdiffGeneralizedHyperbolicgenericsglueherejsonlitekeraskernlabKernSmoothkslatticelifecyclemagrittrMASSMatrixmclustmgcvmulticoolmvtnormnlmenloptrnumDerivpngpracmaprocessxpsquadprogquantmodR6rappdirsRcppRcppArmadilloRcppTOMLreticulaterlangrprojrootRsolnprstudioapirugarchSkewHyperbolicspdtensorflowtfautographtfrunstidyselecttruncnormtseriesTTRvctrswhiskerwithrxtsyamlzeallotzoo
Readme and manuals
Help Manual
Help page | Topics |
---|---|
Hybrid ARMA-LSTM Model for Time Series Forecasting | ARMA.LSTM |